- Home
- Business
- Insights Blog
- Search
Highlights: Businesses continue to face economic and industry changes, making adaptability and resilience crucial [...]
Highlights: Fraud, especially income/employment misrepresentation, is a major concern for auto lenders, who [...]
Highlights: Optimizing consumer loan decisions requires smart, data-driven strategies that adapt to the evolv [...]
Highlights: Fraud prevention, alternative data, and Gen Z financial behaviors were prominent themes in H1 202 [...]
Highlights: Using traditional credit data alone may cause auto lenders to overlook potential borrowers with " [...]
Highlights: Technology partners can help lenders succeed in the evolving small business lending market by int [...]
Highlights: Auto dealers can thrive during economic uncertainty by understanding how market conditions [...]
Highlights: The U.S. economy in June 2025 is characterized by a mix of strong labor market indicators [...]
Highlights: Open banking revolutionizes lending by allowing consumers to securely share bank transaction data, [...]
Highlights: Consumer originations increased year-over-year in several lending categories by February 2025, wi [...]
Highlights: Small business lending saw its biggest jump since 2020 in April, but experts advise caution due to the [...]
Highlights: Traditional credit files offer a limited view of creditworthiness, often excluding "thin file" or "cred [...]
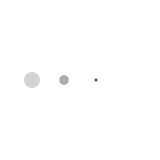